The research, Learning Compositional Neural Programs for Continuous Control, is a collaboration between InstaDeep, Google DeepMind, Sorbonne University and the French National Center for Research (CNRS). It proposes a novel compositional approach to solve robotics manipulation tasks through the solution dubbed AlphaNPI-X, which empirically shows that it can effectively learn to tackle challenging sparse manipulation tasks that require both reasoning and precision, where powerful model-free baselines fail.
Lead author, InstaDeep’s AI Research Engineer, Thomas Pierrot, explains;
“In the research, we formalise the tasks to be mastered as programs, such as computer programs, that are learnt thanks to the Neural Programmer Interpreters (NPI) architecture. The agent learns first simple programs and then learns to compose them together to master more complex ones. We experiment with our method in an environment where the agent controls a robotic arm that faces four blocks with numbers and colours on a table. We show that with this approach, our agent learns to master 20 simple programs such as stack a block on top of another or moving a block to a specific zone. It then learns to compose these together to learn 8 more complex ones such as stacking all the blocks together with colour ordering”.
- Stack all blocks
- Clean the table
- Stack the blocks 1 – 2 – 2
- Clean and stack
Reinforcement Learning for sparse-rewards
While there has been progress in reinforcement learning for tackling increasingly hard tasks, sparse-reward tasks with continuous actions still pose a significant challenge. AlphaNPI-X presents a sample efficient technique for learning in sparse-reward temporally extended settings. You can read more about the research, including the atomic programs and meta-controller execution, in our research section to see how we demonstrate our agent capabilities in a robotic manipulation environment.
AlphaNPI returns to NeurIPS
It is an honour to present the paper at NeurIPS (Advances of Neural Information Processing Systems), a conference widely considered the leading AI conference in the world, as part of the Interpretable Inductive Biases and Physically Structured Learning. The paper is, however, not InstaDeep’s first meeting with the event, nor the first collaboration with Google DeepMind! In fact, AlphaNPI-X builds upon previous AlphaNPI research including ‘Towards Compositionality in Deep Reinforcement Learning’ which was presented on the main stage of NeurIPS 2019, a contribution amongst the top 2.4% of the conference. InstaDeep also participated in 2018 with two research papers and a talk. 2020 sees a record contribution from InstaDeep at NeurIPS with one paper accepted for the conference and four papers accepted for conference workshops, baselines provided for the Flatland challenge and as a co-organiser for a workshop.
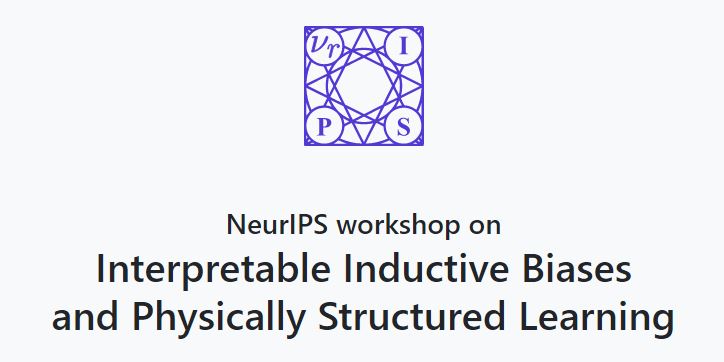
34th Annual NeurIPS
This years’ NeurIPS is the 34rth Annual edition and will be held virtually between 6-12th December. More details and the full program can be found here.
Full list of contributing authors for AlphaNPI-X are:
Thomas Pierrot (InstaDeep), Nicolas Perrin (CNRS, Sorbonne), Feryal Behbahani (DeepMind), Alexandre Laterre (InstaDeep), Olivier Sigaud (Sorbonne), Karim Beguir (InstaDeep), Nando de Freitas (DeepMind).