InstaDeep today announces that it has had three papers accepted for presentation at the 2021 Annual Conference on Neural Information Processing Systems (NeurIPS 2021), including one authored in collaboration with Google Research.
NeurIPS2021 is the 35th edition of the highly prestigious annual machine learning conference, with sessions and workshop tracks presenting the latest research in artificial intelligence and its applications in areas such as computer vision, computational biology, reinforcement learning, and more. InstaDeep has a track record of producing high-quality research in AI, and the acceptance of papers on three different topics validates our in-house research team’s objective of contributing to the global research community.
Overviews of all three papers are below, and registered conference attendees will be able to chat directly with many of the authors about the projects and opportunities at InstaDeep that go into solving the world’s most challenging research problems in decision-making and computational biology, during the event. You can find registration details and the schedule for the all-virtual NeurIPS2021 conference here.
Papers Accepted at NeurIPS2021
On pseudo-absence generation and machine learning for locust breeding ground prediction in Africa
In collaboration with Google Research, we set out to help alleviate the threat to food security in Africa posed by desert locusts by supporting early warning for locust invasion using machine learning (ML). The Food and Agricultural Organization (FAO) of the United Nations has been observing desert locusts since 1985 and they maintain a database of these observations, which contain recordings of locust sightings at different stages of their lifecycle. This type of dataset which only records confirmed sightings is referred to as a presence-only dataset. To train an ML system, there is a need to complement presence data with absence data. The problem of having a presence-only dataset is well known in the domain of species distribution modelling and different techniques have been proposed for generating pseudo-absence data. However, most work on locust breeding ground prediction has resorted to using only random sampling.
As a first step in assisting early warning with ML for locust outbreaks, we tested several methods proposed in the literature for pseudo-absence generation in order to identify which method is most suitable. The methods tested include random sampling (RS), random sampling with environmental profiling (RSEP), random sampling with background extent limitation (RS+) and random sampling with environmental profiling and background extent limitation (RSEP+).
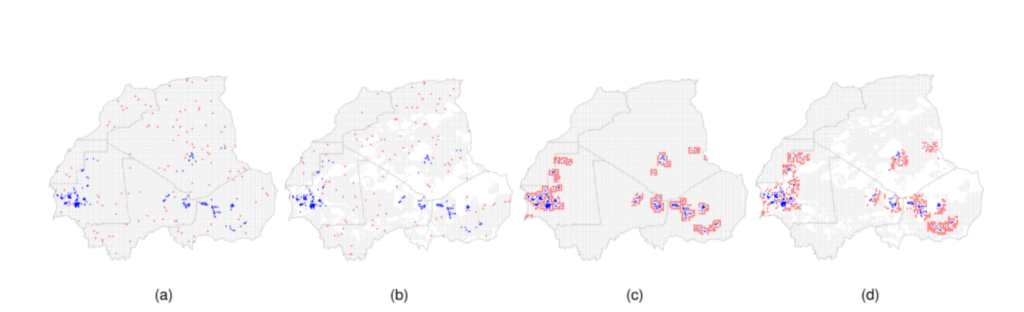
Our study area covered the whole of Africa and we enriched the locust observation data from FAO and pseudo-absence generation with environmental and climatic data from NASA and ISRIC SoilGrids. We found that random sampling with environmental profiling (RSEP) is most suitable when used with logistic regression, outperforming more sophisticated ensemble methods such as gradient boosting and random forests. Our study is one of the first to consider the entire continent with the performance of each tested model measured in terms of its ability to generalise across many different countries.
We envisage future research in using ML for early warning at this scale will drive further insight into how locust breeding ground prediction may be refined and improved. We are excited by the potential of ML assisted systems to extend response lead times for teams on the ground, helping them help others, more effectively.
Links: ArXiv | Artificial Intelligence for Humanitarian Assistance and Disaster Response Workshop | Machine Learning for the Developing World
One Step at a Time: Pros and Cons of Multi-Step Meta-Gradient Reinforcement Learning
Applying Reinforcement Learning to real-life problems is notoriously hard. These algorithms are known for their poor sample efficiency and their brittle convergence properties, which necessitate meticulous hyper-parameter tuning. This tends to yield long and expensive development cycles due to the large compute budget and the time required to train the models. Meta-Gradient Reinforcement Learning has emerged as an appealing solution to this problem, due to its ability to exploit the differentiability of the agent’s learning rule. By automatically tuning its hyperparameters while training, it effectively lets the system learn its own optimal set of hyper-parameters. As a result, the system not only achieves higher quality than before, it also leads to more stable results facilitating the deployment of RL algorithms in production.
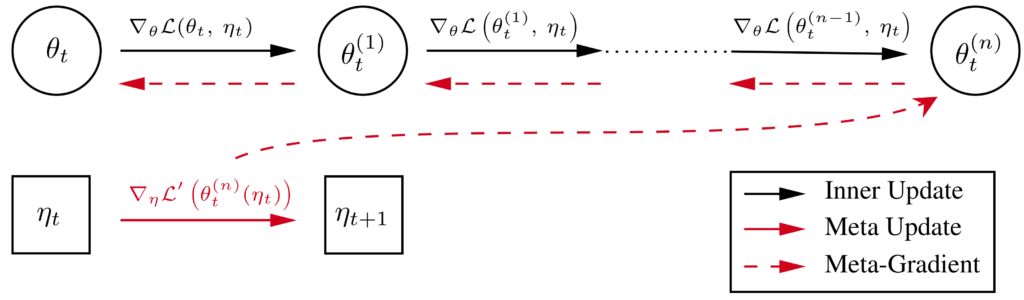
to get θt(n), using the same meta-parameters ηt. The n-step meta-gradient is then computed by
backpropagating through the n gradient steps.
Although online meta-gradient updates have been widely adopted for self-tuning learning algorithms, we find that they suffer from a poor signal-to-noise ratio and a myopic bias that hinder their performance in practice. In this research, we study the impact of using multiple parameter updates in the meta-gradient computation. Despite multi-step meta-gradients being more accurate in expectation, we show that they tend to yield higher variance, limiting their usage in practice. We demonstrate the potential of trading off bias and variance in the way meta-gradients are computed. To this extent, we propose a more robust learning outcome by mixing intermediate parameter updates. This will achieve lower variance while maintaining high performance. By heavily reducing the meta-gradient variance, our method accelerates training and achieves better asymptotic performance in the studied environment.
At InstaDeep, we believe that meta-learning for automatic self-tuning is key to significantly improving deep reinforcement learning, as it has the potential to outperform best human designs. As such, we believe Meta-Gradient Reinforcement Learning will become an essential tool to assist researchers in the development of AI-based decision-making systems and facilitate their deployment. Through this work, we aim to improve our understanding of these methods, to make agents learn with greater efficiency.
Links: ArXiv | Meta-Learning Workshop | extra materials/assets* (*NeurIPS registration required)
Causal Multi-Agent Reinforcement Learning: Review and Open Problems
We live in a causal world, but RL agents don’t necessarily understand the world in a causal way. Though correlation is not causation is well understood, it isn’t embodied by the majority of models we apply in practice. Judea Pearl’s ladder of causation expresses how different types of interaction with an environment limits the types of reasoning an agent can perform. This is a limit experienced by conventional RL agents, which attempt to optimise the reward they receive by selecting optimal actions. This policy optimisation is inherently causal as it requires a delicate understanding of the causal relationships between variables in the agent-environment interface. Though causal RL research is still in its infancy, there are existing use-cases of related methods in causal machine learning already providing value to companies such as Microsoft and Netflix. For example, contextual bandit algorithms are replacing the conventional A/B test in e-commerce and recommendation systems because they add additional benefits of automated counterfactual reasoning. Causal RL has, in theory, shown markedly improved sample efficiency, robustness, and interpretability. These are all important aspects that conventional RL algorithms need to improve upon before they can be fully utilised in the industry.
Previous work in causal RL has looked at the benefits of bridging RL and methods from causality by reformulating RL models in terms of causal models. Generally, this has been attempted with specific tasks in mind, such as off-policy learning, data-fusion, and counterfactual reasoning. In causal inference, a common causal model used for formal reasoning is given by the Structural Causal Model (SCM). Causal Multi-Agent Reinforcement Learning: Review and Open Problems considers extending causal RL ideas to the multi-agent case, where additional complexities arise due to interacting agents. We suggest wrapping and considering MARL models in terms of multi-agent causal models (MACMs), and suggest several areas where this could provide an improvement, including dealing with non-stationarity, communicating in a robust way, and reasoning in a decentralised manner.
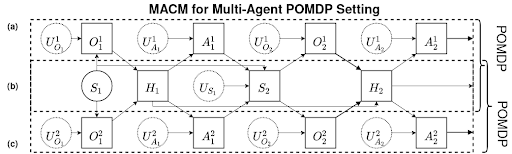
Links: ArXiv | Cooperative AI Workshop | Causal Inference Challenges in Sequential Decision Making: Bridging Theory and Practice Workshop
To register for NeurIPS2021, visit the event registration page.
You can also follow the InstaDeep page on medium.com for regular postings of the company’s research and related materials. Interested in our research work? Please consider joining us! Visit our careers page and advertised openings.